DNA
Genotek’s new material on Geektimes Try to answer everything in order. And yes, we will also define the Jews.
Races aka population groups in biology, medicine and genetics
Mankind has a bad habit of justifying violence by the “inherent” superiority of one race over another – that is why modern biologists are approaching the issue of genetic differences between populations with the care of a sapper. (He), the existence of biological boundaries between racial and ethnic groups was vigorously discussed throughout the 20th century, but a final consensus on this issue has not yet been achieved.1
There were hopes that the sequencing of the human genome of all would be reconciled. The genome, read “from” and “before”, will show that the boundaries between the groups are of a social nature, and the genes are the same for all. It turned out differently: careful study of the human nucleotide code revived and strengthened interest in biological differences between racial and ethnic populations. The same, in general, genes had slightly different allelic variants associated with the risk of diseases (2), the metabolism of drugs (3), the body’s response to environmental conditions (4), and these variants were found in different populations at different frequencies.
The search for non-existent “Indian” or “African” genes was discontinued, but studies in the field of medical and population genetics still draw parallels between the biological characteristics and ethnicity of the participants. The use of the terms “race” and “ethnicity” in such works is actively discussed (and often condemned). There have been attempts to introduce rules that force researchers to justify the need to use “slippery” categories and clarify what exactly is meant by specific terms. In February last year, in Science, one of the most authoritative scientific journals, an ambiguous article (5) appeared, proposing to completely abandon the use of the term “race” in genetic research, replacing it with a more correct and neutral “ancestry” – “origin” .
But even in conditions of uncertainty with terms to divide the humanity into population groups, it is still necessary: in particular, for the correct conduct of clinical trials of drugs and the assessment of the risk of diseases. For example, three allelic variants of the NOD2 gene – R702W, G908R and 1007fs – are associated with an increased risk of Crohn’s disease in Americans of European descent (6.7), however, none of these variants is associated with Crohn’s disease in Japanese (8). There are alleles of the CCR5 gene that affect the rate of development of immunodeficiency in HIV-infected patients (9): among them was found a variant that slows the progression of the disease in Americans of European origin, but accelerates its development in African Americans (10). Asians found a correlation between the polymorphisms of the p53 gene, which regulates the stress response and suppresses the development of tumors, and the medium-winter temperatures in the habitat of populations-the genetic adaptation to frost (11). And if in the past for the division of the sample into ethnic groups only information reported by the participants was used, in the post-genomic era they are increasingly supplemented and refined with a genetic evaluation of the origin of the subject.
Genetic variation between populations
In everyday life we divide people into groups according to appearance or language of communication. Most of the Danes are more alike than each of them – the Italian (that’s a cool visualization with averaged portraits of different nationalities). The Danes and Italians are much closer to each other than each of them – to the inhabitants of sub-Saharan Africa: human phenotypes are clustered according to a geographical pattern. The distribution of genotypes has a similar structure: members of a local group tend to have closer kinship ties than residents of remote areas, and populations inhabiting one region are closer than those whose habitats are separated by geographic barriers (for example, a mountain range or water Array).
In this case, the genetic diversity of the human population is lower than in many biological species. This is explained by the fact that humanity is a young species: the individual groups had relatively little time to accumulate differences. Two randomly chosen people differ from each other by ~ 1000 nucleotides, whereas two chimpanzees do not coincide once in ~ 500 “letters”. And, nevertheless, in the total in the human genome there are about 3 million potential “points of divergence”. Most of these inconsistencies, called single nucleotide polymorphisms (SNP), are neutral or almost neutral, but some of them are responsible for phenotypic differences between people.
Distribution of neutral polymorphisms (since they do not carry biological meaning, they are not subjected to directed evolutionary selection, they are carried by the wind of migrations) in the world population reflects the demographic history of our species. Genetic and archaeological data indicate that in the last 100,000 years the size of the human population has increased significantly. People settled outside Africa, colonizing the rest of the world. The process of settlement influenced the geographical distribution of alleles in two ways: firstly, the “founder effect” had an effect – in the migrant population, as a rule, only a part of the genetic variants from the entire pool of their diversity in the ancestral population were represented; Secondly, there was a so-called “assortative crossing”, i.e. Couples were formed predominantly within their group, which restricted the spread of existing and emerging de novo polymorphisms among individuals inhabiting different geographic regions. These processes led to a gradual accumulation of genetic differences.
In the context of population groups, genomic markers began to be studied in the 70s – 80s, in the 90s they were used to identify the population belonging to a particular person. Researchers again and again demonstrated that genetic polymorphisms can successfully isolate population groups and determine the group affiliation of the individual. At the same time it was shown that people living on the same continent tend to be closer to each other genetically than people from different continents. Initially, in such studies, information about the place of birth, race, ethnic group was known from the very beginning and was used in conjunction with genetic data; If the subjects were distributed blindly on clusters, exclusively on the basis of genetic traits, the correspondence between geographic origin, ethnicity and population structure was less pronounced. As further studies showed, success depended on the genetic markers used and their number (more – better), the correct choice of reference populations and other factors (12).
By 2004, the genetic definition of population in the United States was used not only in biomedical research but also in crime investigations: this article from Nature contains a fascinating story about how policemen, desperate to find a criminal, ordered a DNA test In a commercial company, decided on the color of the suspect’s skin and disclosed the case. Proposals on the analysis of genetic origin successfully fell into the wave of general interest of people to their own past. “Roots mania”, this is the name of this fascination in the article in Time, devoted to “America’s latest obsession” – genealogical research.
Experts who use the genomic methods to study the origin and evolution of peoples. For example, in 2013 an international team of researchers used genetic analysis to refute the hypothesis of the origin of Ashkenazi Jews from the Khazars (13). The set of genomic data used by the authors is in the public domain: it contains more than 100 world populations. We propose to work with us to simulate a small study: to determine the location of Genotek customers in this sample, and at the same time to understand the technical details of determining the population population.
The purpose of the study
Identify the location of Genotek customers among the reference populations. To find out whether there are representatives of the Ashkenazim Jews in our sample. Demonstrate principles and methods for analyzing the population belonging to an individual.
Research problems
To process the genotyping data of 722 subjects by the ADMIXTURE program, using as a training sample a set of data from the work of Behar et al., 2013.
Materials and Methods
The initial work of Behar et al., 2013, used the data of 1,774 people: among them were representatives of 88 non-Jewish populations (from Arabia, Central Asia, East Asia, Europe, Middle East, North Africa, Siberia, South Asia and sub- Saharan Africa) and 18 Jewish populations. An extensive set of data was needed for the authors to accurately determine the location of Ashkenazi in the context of world populations: the task was to present all three geographic regions from which this group could hypothetically originate – Europe, the Middle East and the Khazar Khaganate. The authors emphasized the difference between the approach to the selection of samples representing modern European, Middle Eastern and Jewish populations – direct descendants of ancestral populations, and samples corresponding to the Khazar Khaganate, which ceased to exist approximately 1000 years ago. The catch is that none of the currently existing populations is a direct heir of the Kaganate. The authors selected the inhabitants of the South Caucasus (Abkhazians, Armenians, Azeris, Georgians), the North Caucasus (Adygs, Balkars, Chechens, Kabardins, Ossetians and several other nationalities) as Chuvs as possible modern representatives of the Khazars, Chuvash and Tatars.
We added to the data set samples of 722 people from various regions of Russia.
For the statistical analysis the program ADMIXTURE was used, which allows to estimate the most probable origin of the individual on the basis of data on genotypes. In addition to it, the authors of the article in question used other statistical methods that gave a similar answer to the question posed. We will dwell on ADMIXTURE, since it is this algorithm that allows estimating the percentage contribution of ancestral populations to the studied genomes.
ADMIXTURE uses Monte Carlo methods in Markov chains (Markov chain Monte Carlo, MCMC). Here is a link to the article by the authors of the algorithm for those who want to understand more in detail the mathematical side of the process.
Let’s consider how ADMIXTURE works on the example of samples and populations from our set
In total, we have 2,496 specimens / individuals, each of which belongs to one of 106 modern populations. We assume that modern populations are most likely derived from a relatively small number of ancestral populations. “Ancestral populations” in this analysis are some ancient genomic clusters, united by the principle of genetic similarity. ADMIXTURE allows both arbitrarily to make assumptions about the number of such clusters in the sample, and to select the optimal number of them, most correctly describing the real distribution of genomic data.
Having obtained information about the genotypes and the estimated number of “ancestral” populations (K), ADMIXTURE builds a model that estimates the contribution of each of the “ancestral” populations to each sample. In the interpretation of data, both the quantitative composition of the genome (the percentage of clusters) and the qualitative one (their presence or absence in specific genomes) is important. Based on these data, it is possible to make assumptions about evolutionary processes in the population, in particular, about the presence or absence of common “roots” in population groups. However, the conclusions will be legitimate if the model we have constructed is good: the optimal value of K has been chosen
We select the optimal value of K
How to determine how many “ancestral” populations most closely correspond to the true for a given sample? Empirically!
ADMIXTURE – smart program: building a model of the genetic structure of populations based on data on the genotypes of individuals (estimating the contribution of each of the ancient genomic clusters to each of the sample genomes) for a given number K, it does not forget to make a comparison with reality at the end. Check how well the input data is described by the constructed model. The measure of comparison is “error” – a value describing the discrepancy between the model and real data. The larger the error, the worse the assumption of the number of ancestral populations corresponds to reality.
How to choose the optimal value of K? We start the ADMIXTURE algorithm on this sample, substituting different values of K, and for each K we get our error value. We plot the dependence of the magnitude of the error on K. This is the graph obtained by the authors of the article:
The optimum value of K is at the minimum of the function. If the minimum on the graph is not found (the function is constantly increasing or decreasing), it will be necessary to build models, choosing new K, until you can find the right one.
Even with optimally selected K, the reliability of the analysis results depends on the correctness Of the sample:
1. Individuals should not be related to each other.
2. Single nucleotide polymorphisms (SNP), for which genotyping is performed, should be evenly distributed across the genome with a sufficiently high density.
3. Alleles of SNP should be in equilibrium coupling, that is, the probability of having a given allele in a particular individual should depend only on the frequency of this allele in the population, but not on other alleles in the genome.
As seen from the graph, the optimal K For this sample was 10 “ancestral” populations.
Results
The results of the analysis of ADMIXTURE are visualized like this:
Each cluster has its own color, and the populations differ (or do not differ) in the shares of clusters in the genome. Here is an interactive version of the picture for a detailed study: move the mouse and scroll to see all the populations or consider one of the groups in more detail.
In general, within the “population” of Genotek, the cluster ratio is expected to match the pattern characteristic for Populations of Eastern European origin. Interesting starts at the level of individual samples:
Although the population closest to the given sample is determined from numerical values, a lot of information can also be obtained by visual comparison of the patterns. We suggest you to determine the closest populations for the samples of four Genotek clients from the picture yourself.
In this picture, samples 1 and 2 are of Asian origin: the predominance of the pink cluster is characteristic of the Japanese and of the Khan in our sample, the blue one for the Yakuts, the third sample shows the ratio Components, typical for Russians, Byelorussians, Ukrainians and Poles, and the fourth is a typical Ashkenazi Jew.
In total, among the 722 samples, we found nine Ashkenazi Jews.
Conclusion
Population affiliation is by no means the only factor determining the ethnic self-identification of a person. However, it is still possible to identify the correlation between ethnic groups and the structure of the genome of their representatives. Such analysis is used both for scientific and medical purposes, and for studying its own roots by all comers. It is important to understand that the models are constantly being improved, and the results obtained for greater accuracy should be considered together with other data, for example, the family genealogical tree.
The authors of the original article did not find evidence of the Khazar origin of Ashkenazi. Genetic tests, of course, “know how” to identify the Jews – but do not forget that “Jewry” – is primarily a state of mind.
Genotek will launch an updated Genealogy DNA test in the near future with extended results: we will bring the number of populations to a hundred, we will add Jewish populations. We will update the information in the personal account for all who have ever handed over their genetic material to us. Если вы все еще не отгенотипированы, приглашаем присоединиться.
Список литературы
- Foster M., Sharp R. (2002). Race, Ethnicity, and Genomics: Social Classifications as Proxies of Biological Heterogeneity. Genome Res.
- Collins F.S., McKusick V.A. (2001). Implications of the Human Genome Project for medical science. JAMA.
- Nebert D.W., Menon A.G. (2001) Pharmacogenomics, ethnicity, and susceptibility genes. Pharmacogenomics J.
- Olden K., Guthrie J. (2001). Genomics: Implications for toxicology. Mutat. Res.
- Yudell M., Roberts D., DeSalle R., Tishkoff S.(2016). Taking race out of human genetics. Science.
- Ogura, Y. et al. (2001). A frameshift mutation in NOD2 associated with susceptibility to Crohn’s disease. Nature.
- Hugot, J. P. et al. (2001). Association of NOD2 leucine-rich repeat variants with susceptibility to Crohn’s disease. Nature.
- Inoue, N. (2002). Lack of common NOD2 variants in Japanese patients with Crohn’s disease. Gastroenterology.
- Martin, M. P. et al.(1998). Genetic acceleration of AIDS progression by a promoter variant of CCR5. Science.
- Gonzalez, E. et al.(1999). Race-specific HIV-1 disease-modifying effects associated with CCR5 haplotypes. Proc. Natl Acad. Sci. USA.
- Shi, Hong et al. (2009). Winter Temperature and UV Are Tightly Linked to Genetic Changes in the p53 Tumor Suppressor Pathway in Eastern Asia. American Journal of Human Genetics.
- Bamshad M., Wooding S., Salisbury B. et al. (2004). Deconstructing the relationship between genetics and race. Nat Rev Genet.
- Behar D.M. et al. (2013). No Evidence from Genome-Wide Data of a Khazar Origin for the Ashkenazi Jews. Human Biology.
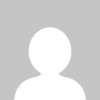
Saved as a favorite, I really like your site!
I have read several good stuff here. Definitely price bookmarking for revisiting.
I wonder how much attempt you place to create one of these
magnificent informative website.
First off I would like to say fantastic blog! I had a quick question in which I’d like to ask if you don’t mind. I was curious to know how you center yourself and clear your thoughts before writing. I’ve had difficulty clearing my thoughts in getting my thoughts out. I do take pleasure in writing but it just seems like the first 10 to 15 minutes tend to be wasted just trying to figure out how to begin.
I all the time used to read post in news papers but now as I am a user of internet therefore from now I am using net for articles, thanks to web.
Definitely believe that which you stated. Your favorite justification appeared to be on the internet the easiest thing to be mindful of. I say to you, I definitely get irked while people think about concerns that they plainly do not understand about. You managed to hit the nail upon the top and also defined out the entire thing with no need side-effects , other folks can take a signal. Will likely be back to
I do accept as true with all of the ideas you’ve introduced on your post. They are really convincing and will certainly work. Still, the posts are too brief for starters. May just you please extend them a little from next time? Thanks for the post.